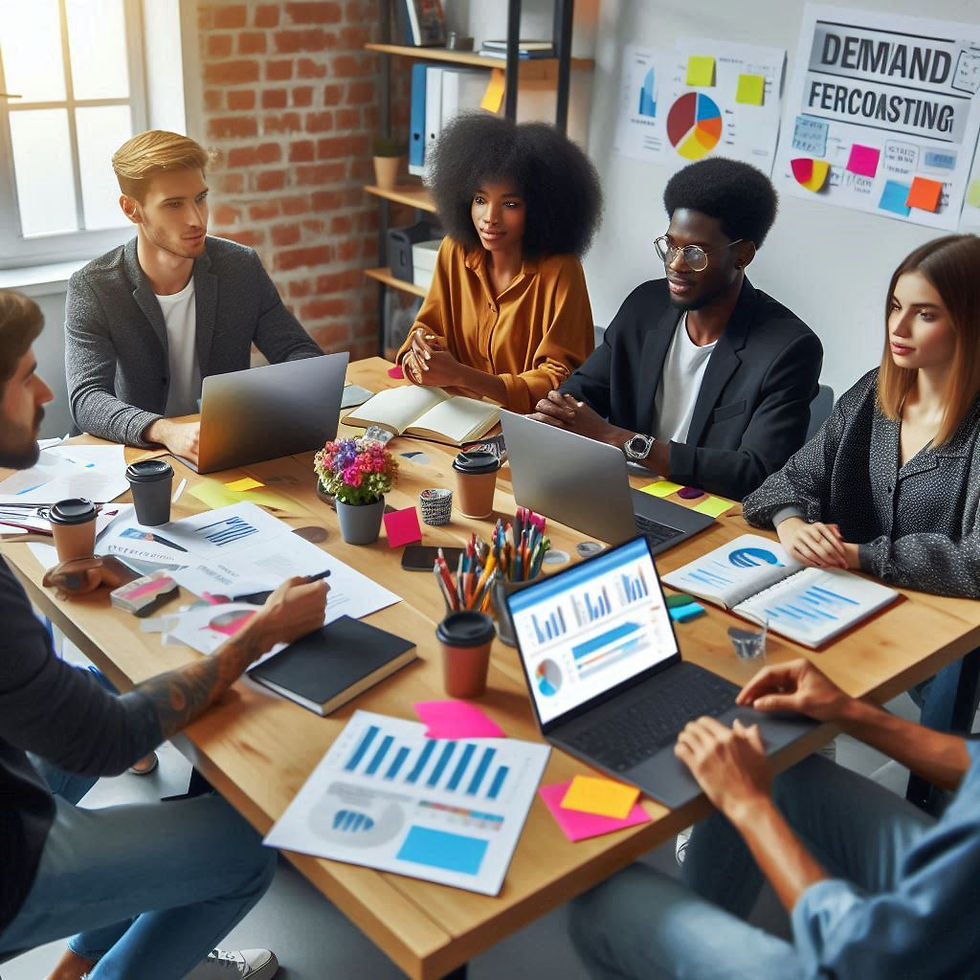
1. What is Demand Forecasting?
Demand forecasting is the process of predicting future customer demand for a product or service based on historical data, market trends, and other relevant factors. This practice is essential for businesses to plan and manage their supply chain, inventory, production, and marketing strategies effectively. By leveraging statistical analysis, machine learning algorithms, and qualitative assessments, demand forecasting helps businesses anticipate customer needs and adjust their operations accordingly. The goal is to strike a balance between supply and demand, minimize costs, and maximize customer satisfaction.
2. Why is Demand Forecasting Important?
Demand forecasting is crucial for several reasons:
Inventory Management: Helps maintain optimal inventory levels, reducing the risk of overstocking or stockouts.
Production Planning: Informs production schedules to ensure that supply meets anticipated demand, avoiding wastage and inefficiencies.
Financial Planning: Provides insights into future revenue and cash flow, supporting budgeting and financial planning.
Resource Allocation: Aids in the efficient allocation of resources, such as labor, materials, and capital.
Customer Satisfaction: Ensures that products are available when customers need them, enhancing customer satisfaction and loyalty.
Strategic Decision-Making: Supports strategic decisions related to pricing, marketing, and expansion based on expected demand.
Cost Reduction: Minimizes costs associated with excess inventory, emergency production runs, and expedited shipping.
In essence, demand forecasting helps businesses anticipate and prepare for future customer needs, enabling them to optimize operations, reduce costs, and improve overall efficiency.
3. When to Use Demand Forecasting?
Demand forecasting can be applied in various scenarios, particularly when:
Launching New Products: To predict the demand for new products and plan production and inventory accordingly.
Seasonal Planning: To anticipate changes in demand due to seasonal trends and adjust inventory and production schedules.
Promotional Campaigns: To estimate the impact of marketing and promotional activities on demand.
Supply Chain Management: To optimize supply chain operations and ensure a balanced flow of goods.
Capacity Planning: To plan for future capacity needs in production, warehousing, and distribution.
Strategic Planning: To integrate demand insights into broader strategic planning and decision-making processes.
Anytime there is a need to anticipate future customer demand to optimize operations and strategic planning, demand forecasting should be employed.
4. What Business Problems Can Demand Forecasting Solve?
Demand forecasting can address several business challenges:
Stockouts and Overstocks: Predicting demand accurately helps avoid stockouts (leading to lost sales) and overstocks (leading to excess inventory costs).
Inefficient Production Schedules: Ensuring production schedules align with demand to avoid underproduction or overproduction.
Inaccurate Financial Planning: Providing reliable demand estimates to support accurate financial forecasting and budgeting.
Ineffective Marketing Strategies: Informing marketing strategies based on expected demand, optimizing promotional efforts.
Supply Chain Disruptions: Anticipating demand fluctuations to minimize disruptions in the supply chain.
Resource Wastage: Reducing wastage of resources by aligning production and inventory levels with demand.
5. How to Use Demand Forecasting?
Using demand forecasting effectively involves several steps:
Define Objectives and Scope:
Identify Goals: Determine what you aim to achieve with demand forecasting, such as optimizing inventory levels, improving production planning, or enhancing financial forecasting.
Specify Scope: Define which products, services, or markets will be included in the forecast.
Data Collection and Preparation:
Gather Data: Collect historical sales data, market trends, economic indicators, and other relevant data.
Clean Data: Ensure data quality by cleaning and preprocessing the data to remove errors, inconsistencies, and duplicates.
Transform Data: Transform the data into a suitable format for analysis.
Choose Forecasting Methods:
Qualitative Methods: Use expert opinions, market research, and Delphi method for new products or when historical data is limited.
Quantitative Methods: Use statistical models such as moving averages, exponential smoothing, ARIMA, and machine learning algorithms for more accurate forecasts.
Build Forecasting Models:
Select Algorithms: Choose appropriate algorithms for the chosen forecasting methods.
Train Models: Train models using historical data to identify patterns and make predictions.
Validate Models: Validate the models to ensure their accuracy and reliability.
Analyze and Interpret Results:
Interpret Forecasts: Interpret the forecasted demand to gain actionable insights.
Understand Implications: Understand the business implications of the forecasts and how they can inform decision-making.
Develop Action Plans:
Create Strategies: Develop actionable strategies based on the forecasted demand, such as adjusting inventory levels, modifying production schedules, or reallocating resources.
Set Priorities: Prioritize actions based on their potential impact and feasibility.
Implementation and Monitoring:
Execute Plans: Implement the action plans, ensuring that all necessary resources are in place.
Monitor Progress: Continuously monitor the accuracy of the forecasts and the impact of the changes.
Feedback and Adjustment:
Gather Feedback: Regularly gather feedback from stakeholders to assess the effectiveness of the implemented changes.
Adjust Models: Make necessary adjustments to the forecasting models and action plans based on feedback and ongoing analysis.
6. Practical Example of Using Demand Forecasting
Imagine you are the operations manager for a consumer electronics company and you want to use demand forecasting to optimize inventory levels for the upcoming holiday season.
Define Objectives and Scope:
Objective: Optimize inventory levels for the holiday season.
Scope: Analyze data specific to popular consumer electronics products.
Data Collection and Preparation:
Gather historical sales data for the past holiday seasons, market trends, and economic indicators.
Clean the data to remove errors, inconsistencies, and duplicates.
Transform the data into a suitable format for analysis.
Choose Forecasting Methods:
Quantitative Methods: Use time series analysis and machine learning algorithms for accurate forecasts.
Build Forecasting Models:
Select algorithms such as ARIMA and random forest regression.
Train the models using historical sales data to identify patterns and make predictions.
Validate the models to ensure their accuracy and reliability.
Analyze and Interpret Results:
Interpret the forecasted demand to determine the expected sales for each product during the holiday season.
Understand the business implications of these forecasts, such as the need for increased inventory levels for high-demand products.
Develop Action Plans:
Create strategies to optimize inventory levels, such as increasing stock for high-demand products and adjusting reorder points.
Develop initiatives to ensure timely restocking and efficient warehouse operations.
Set priorities based on the potential impact of these actions.
Implementation and Monitoring:
Execute the action plans, ensuring all necessary resources are in place.
Monitor the accuracy of the forecasts and the impact on inventory levels through regular tracking.
Feedback and Adjustment:
Gather feedback from sales and warehouse teams to assess the effectiveness of the changes.
Adjust the forecasting models and action plans based on feedback and ongoing analysis to ensure continuous improvement.
7. Tips to Apply Demand Forecasting Successfully
Engage Stakeholders: Involve key stakeholders from sales, marketing, finance, and operations to ensure a comprehensive analysis.
Use Reliable Data: Ensure the data collected is accurate and up-to-date to make informed decisions.
Leverage Technology: Utilize advanced analytics tools and software to automate data collection, analysis, and visualization.
Focus on Key Metrics: Identify and focus on the key metrics that are most relevant to your forecasting goals.
Iterative Approach: Adopt an iterative approach to demand forecasting, continuously refining models and strategies based on new insights.
Communicate Clearly: Clearly communicate the findings and action plans to all relevant stakeholders to ensure buy-in and support.
Scenario Planning: Consider different scenarios and their potential impacts to build robust and flexible forecasts.
8. Pitfalls to Avoid When Using Demand Forecasting
Ignoring Data Quality: Using inaccurate or incomplete data can lead to misleading forecasts.
Overfitting Models: Creating models that are too complex can result in overfitting, making them less generalizable to new data.
Assuming Static Demand: Recognize that demand can be dynamic and influenced by various factors over time.
Neglecting to Monitor: Not monitoring the accuracy of forecasts and their impact can result in not achieving the desired outcomes.
Resistance to Change: Failing to manage change effectively can lead to resistance from employees, hindering the implementation of action plans.
Focusing Only on Short-Term Gains: Balancing short-term improvements with long-term strategic goals is crucial for sustainable success.
Not Taking Action: Conducting the analysis but failing to implement the findings leads to wasted effort and missed opportunities.
By following these guidelines and avoiding common pitfalls, you can effectively use demand forecasting to anticipate customer needs, optimize operations, and drive business success.
Comments